At Amalgam Insights, we’ve long championed the Technology Lifecycle Management approach to describe the many areas existing across IT FinOps to reduce costs across hyperscalers, data clouds, Software-as-a-Service, networking, enterprise mobility, and telecom costs. Usage optimization is not enough. Product rationalization is not enough. Contract negotiations are not enough. And when these opportunities are not bridged, additional opportunities fall by the wayside across the IT environment.
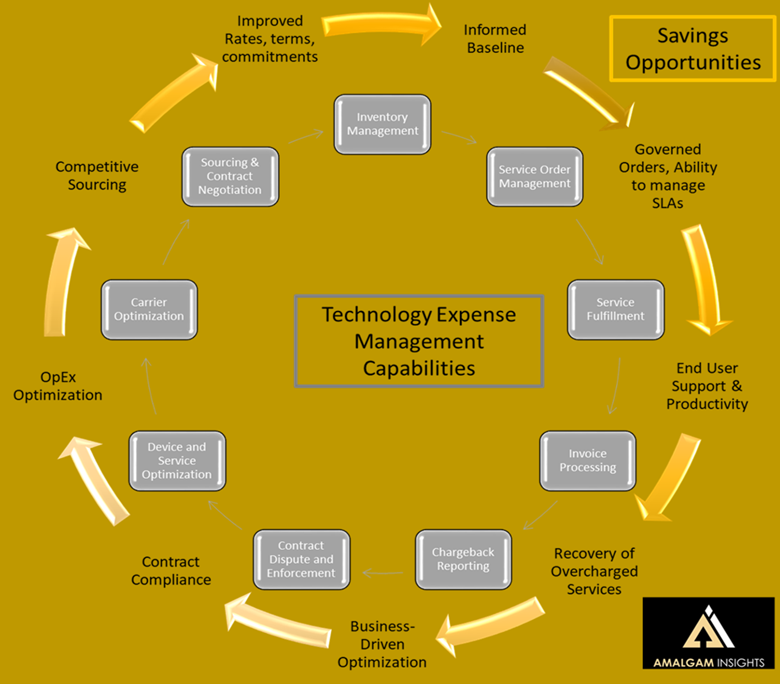
One of the most interesting trends of 2024 has been the FinOps Foundation’s initial steps into software management. As cloud FinOps starts entering the already-established worlds of SaaS management and network management, it will be interesting to see both where cloud FinOps’ comfort zone of massive usage and product tradeoffs help the more static worlds of software and telecom as well as where FinOps’ traditional struggles with business taxonomy, detailed sub-user chargebacks, and lack of contract detail start to push against the issues that you, I, and the rest of the IT world have been dealing with for the past 15+ years. Unlike Cloud FinOps, Software FinOps and Telecom FinOps have a long history of multi-user monitoring, usage, and cost management and to avoid cooperating with end-user requests for any type of market-wide billing or usage standardization.
For the past several months, I’ve been working on my SmartList of IT FinOps vendors (thank you to all the vendors and customers for your participation!) with a eye towards looking at vendors focused on bridging gaps between cloud FinOps and the rest of the IT world. Out of the 300+ vendors I look at from an IT expense lifecycle perspective, I’ve narrowed the list down to 15 vendors that I think are really doing something different, which will be our Distinguished Vendors. But I am also starting to find some interesting trends that make these distinguished vendors different from the extremely crowded portfolio of options that IT departments face.
Before getting to those, let’s just define what is not considered differentiated in 2024. Simply ingesting bills is not good enough. Being customer-centric and having attention to detail are considered table stakes if you cannot provide customers that vouch for your approach being quantitatively better than other competitors. Everybody I cover can support tagging. Cross-charging is a standard enterprise ask in RfX activity. Basic dashboards and analytics are standard (and if you haven’t chosen a high-performance analytics solution like Sisense or Sigma or Qrvey, you’re probably behind for supporting real-time changes). And everyone is competing against 2020s-era design standards for SaaS as a standard, especially with the flood of money that has gone into the data and code-centric Third Wave of FinOps.
First, as expected, top vendors are often finding now that they are the second or third solution chosen at the enterprise level. IT buyers have often focused on the “up and to the right” vendor landscape too much in their purchase of FinOps and IT expense management solutions with the assumption that these are commoditized solutions rather than the financial window to the pulse of the digital business. Although every business today likes to talk about how they have “digitally transformed” and are “data-driven,” the truth is that companies have not fully embraced data and digital business until the actual cost of data infrastructure, delivery, and data-related customer activities can be finally categorized and calculated. And without FinOps and IT expense solutions, the comparative data simply isn’t there at the bill of material (BOM) or component level that we take for granted in manufacturing or service level. A digital business lacking formal IT FinOps is like managing a hospital without knowing how many procedures are being done or a discrete manufacturing company not knowing how many parts it uses.
Second, there is a massive effort to utilize and translate tagging efforts into standard business categories. The FinOps insistence on tagging service elements across infrastructure and platform without alignment to larger corporate or IT frameworks is starting to collapse into its own form of technical debt. The combination of poor data quality, inconsistent or idiosyncratic tagging, poor mapping to existing categories, and lack of integration with existing birthright and foundational enterprise applications has led to the need for better metadata definition and mapping. Of course, the goal here is not simply to create a pristine metadata environment (although I do love well defined taxonomies and ontologies!), but to deeply align IT to business so that product, service, and revenue managers know which technologies are vital to their work.
Third, data integrations and a unified data layer are increasingly important across vendors. One of the most salient and welcome aspects of the latest wave of FinOps solutions is the data layer that provides visibility across hyperscalers, data clouds such as Databricks, Snowflake, and Cloudera, as well as observability solutions such as Datadog. From a tactical perspective, this data ingestion helps cloud architects and digital business leaders to track costs. But it also helps set up the FinOps practitioner as a central player in managing the business aspects of digital behavior. The cost of accessing, processing, and utilizing data will only increase with the emergence of AI as a new massive spend area within IT. And although this cost is under the direct supervision of the CTO and CFO right now in many cases, it will all eventually filter down to some level of IT FinOps as the complexity of these ecosystems becomes too immense. Many of today’s top solutions are proactively setting themselves to be this data layer for supporting the future of IT activity.
Fourth, the tradeoffs between defined hyperscalers, defined on-premises services, virtualized services, and containerized services continue to be a challenge and the current answer is often to split each of these categories into a separate management solution. This is not a sustainable approach and Amalgam Insights both expects and encourages market consolidation so that FinOps professionals can see more of their infrastructure and platform investments under the same umbrella.
Fifth, everybody likes to talk about “cloud economics” while ignoring the “economics” part of the puzzle. There is a lot of focus on the mathematical aspects of large scale optimization of usage. And I get it. Techies love working with big problems and algorithmic fixes and finding clever ways to discover null values and aggregating pennies into dollars. This skill set is often what makes technology contributors invaluable both in developing architecture and in root-cause analysis for large enterprise challenges. But it is only a piece of the puzzle when it comes to aligning the financial health of the enterprise with digital and cloud activity. At some point, this optimization needs to be tied to the less exciting tasks of invoice processing, cross-checking billing rates with existing rates and discounts, direct product tradeoffs both within and across hyperscaler providers, comparing contract terms, enforcing contracted KPIs and timeframes, and aligning cloud activity to new accounting rules and tax credits. All of this is part of the microeconomics of cloud. Simply looking at usage optimization makes you a unit-specific beancounter. A true cloud economist needs to understand how the cost of cloud shapes the rest of the business and how it needs to be structured, reinforced, and defined based on all business forces.
Sixth, the cost of IT FinOps is changing rapidly. The first generation of cloud FinOps solutions charged similarly to software management and telecom management vendors where enterprise spend was based on a percentage of spend. But the problem is that these approaches started to outgrow their value for clients that grew their cloud spend to $50 million or $100 million and literally realized they could build their own solution and support it in perpetuity. We have seen solutions like Capital One Slingshot or Netflix’s development of NetflixOSS Ice, now supported by Teevity based on corporate efforts that became commercial products. So, there is a lot of pressure to drive down the cost of IT FinOps back to a flat license rate or user-based pricing once spend gets to an enterprise-tier. This pressure is exacerbated by the vendor sprawl across FinOps. For vendors that are product-based, this will continue to be a trend while companies shifting to a managed services approach will be able to maintain pricing based on the overall maintenance of the FinOps environment across billing, accounting, optimization, procurement, vendor relationships, governance, and IT-business alignment.
Those are some of the big trends I’ve been seeing so far over the last several months. There is a lot of nuance here, which I’m looking forward to digging into as I finalize the FinOps SmartList and get to highlight the Distinguished Vendors: the top 5% of companies that stand out both in their results and their differentiation. Is there anything you think I missed? Or do you want to talk more about either your FinOps product or your current FinOps RfP work? If so, get in touch with me at briefings@amalgaminsights.com.